
In particular, we present a chance-constrained optimization for Stochastic Discrete-time Linear Complementarity Systems (SDLCS). Keywords: Robotics, Optimization, Stochastic optimal controlĪbstract: This paper presents a chance-constrained formulation for robust trajectory optimization during manipulation. Mitsubishi Electric Research Laboratories We found that our pipeline was effective at imitating the simulated behaviors using both accurate and inaccurate assumptions, being able to closely identify not only the policy gains, but also the agent’s radius of communication and their maximum velocity constraint.Ĭhance-Constrained Optimization in Contact-Rich Systems To show our approach’s ability for imitating swarm behavior, we apply this methodology to both simulated and physical swarms (i.e., a school of fish) exhibiting a multitude of distinct emergent behaviors. The trajectory data of known swarms is used with linear regression and nonlinear optimization methods to learn the relative weight of each policy. Agent-level controllers are treated as a set of policies that are combined to dictate the agent’s change in velocity. This paper proposes a novel method to circumvent this problem by learning the agent-level controllers of an observed swarm to imitate its emergent behavior. Currently, there is no known swarm control algorithm that maps global behaviors to local control policies. Imitating Swarm Behaviors by Learning Agent-Level ControllersĪbstract: A main challenge in swarm robotics is the unknown mapping between simple agent-level behavior rules and emergent global behaviors. Different experiments are shown to demonstrate the algorithm effectiveness. The proposed algorithm permits to finely control the position of the gripper fingers, exploiting a quadratic-programming function to minimize the controller output. Therefore, this letter intends to deliver a new control algorithm for the control of pneumatic grippers. The poorness of the control strategies for the grasping force, as well as for the fingers position, does not match the great advancement that robotics obtained in the last years.
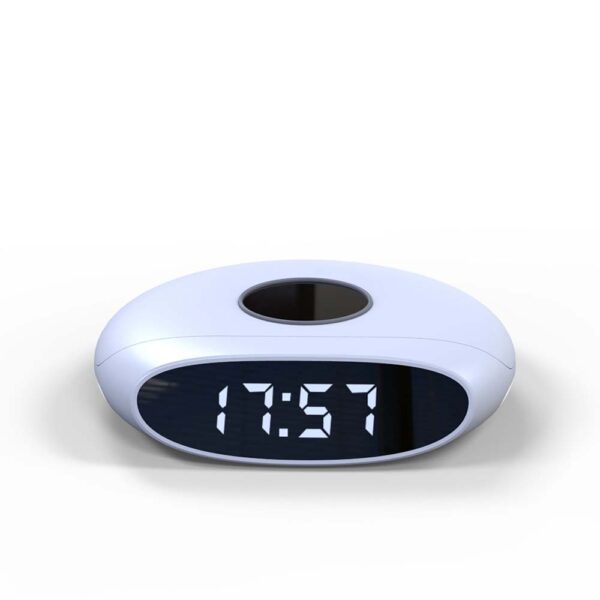
Pneumatic grippers mounted on industrial robotic arms are commonly rigid as high forces might be required during the grasping operations.

Despite the very large employment, pneumatic grippers do not yet offer performance beyond the open/closed behavior in the majority of cases. Keywords: Robotics, Optimization algorithmsĪbstract: Industrial grippers are generally either electric or pneumatic, with the latter being preferred as their air- based functioning guarantees many advantages such as cost effectiveness and reduced encumbrance. Machine Learning for Sparse Nonlinear Modeling and ControlĪ Quadratic-Programming Approach for the Real-Time Control of the Fingers Position in Industrial Pneumatic Grippers Autonomy for Active Perception by Robot Swarms
